Redefining Machine Intelligence: Krishna Kumar’s Vision for Common Sense AI Using Semantic Networks and Dimensionality Reduction
By Impact Desk | Updated: May 26, 2025 19:48 IST2025-05-26T19:45:19+5:302025-05-26T19:48:47+5:30
Introduction: The Pursuit of Human-Like Intelligence in AI In a world where artificial intelligence is transforming industries, one basic ...
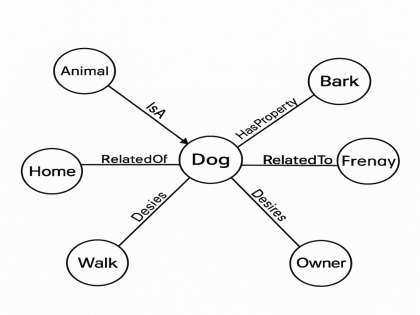
Redefining Machine Intelligence: Krishna Kumar’s Vision for Common Sense AI Using Semantic Networks and Dimensionality Reduction
Introduction: The Pursuit of Human-Like Intelligence in AI
In a world where artificial intelligence is transforming industries, one basic question still goes unasked, can machines really see the world as humans see it? Even though AI programs have come an incredibly long way in language, image recognition, and predictive analysis, they are still weak on tasks that depend on common sense reasoning. It is here in this valued space that Krishna Kumar, as a Senior Product Manager and expert in data and analytics and Artificial Intelligence and Machine learning has succeeded in offering a revolutionary paper through his newly launched research work, "Eigenvector Methods for Common Sense Knowledge Representation: Advances in Semantic Networks and Dimensionality Reduction."
With more than seven years of experience in constructing data products and eight AI and machine learning peer-reviewed research papers, Krishna Kumar brings a unique balance of business acumen and scholarly gravity. His contribution doesn't solve merely a technical problem; it aims to redefine how machines learn and reason about everyday human knowledge.
The Innovation: Eigenvector-based Knowledge Structuring using ConceptNet
At the center of this book is a very strong idea: using mathematical methods such as Singular Value Decomposition (SVD) to organize and reduce enormous, cumbersome collections of natural language data into intelligent semantic networks. Those networks such as ConceptNet, an open-source effort that formalizes commonsense relationships between concepts—underlie AI systems that hope to reason with subtlety, flexibility, and context. Classical approaches, relying on hand-coded rules or naive co-occurrence statistics, tend not to be able to handle the nuances of language and inference. Krishna Kumar's research revives and augments the Analogy Space paradigm using strong eigenvector-based techniques, allowing machines to learn more profound relationships, remove noise, and predict implicit links from knowledge graphs.
Dimensionality Reduction: Making Sense of Sparse, Noisy Data
The significance of this research is deep. Taking advantage of the organization of concepts and relations in low-dimensional space, this research allows AI systems to form analogies, resolve word sense, and even identify emotional tone in text albeit without scaling well across large-scale sparse and noisy data. In contrast to rule-based systems, which fare badly on exceptions, this method learns statistically from data using eigenvectors to capture the character of large-scale human knowledge.
Methodology: Merging Structured and Unstructured Data
Krishna's approach is as utilitarian as it is innovative. His system combines data from structured forms, unstructured user input, and even interactive web games to build a dynamic and ever-changing commonsense knowledge base. By using normalization and filtering on the data before carrying out dimension reduction, his approach provides high-quality reasoning with negligible noise that is irrelevant. This enables the AI to make logical deductions like connecting "keyboard" to "motherboard" or that "table" would have a closer connection with "dining room" than with "newspaper article."
Applications: Bridging Research and Real-World Impact
Most strikingly perhaps, this work transcends theory in academia. Its applications in the real world are already being felt in areas such as semantic search, recommendation systems, sentiment analysis, and real-time inference of concepts. In research like AffectiveSpace and Divisi, Krishna demonstrates how this approach can bridge the gap between affect detection and computational reasoning—abilities essential to next-generation AI systems that must interact with users in a natural way.
Identifying Challenges: Towards Hybrid Models of Reasoning
While the paper advocates for the advantages of SVD-based reasoning, its shortcomings are also discussed freely. Directional problems in relations, sparsity in data, and the difficulty of reasoning with highly novel concepts are discussed freely. Krishna suggests careful directions for the future, e.g., leveraging SVD interpretability and deep learning model expressiveness like transformers or graph neural networks. His vision is distinct: a world where hybrid systems combine symbolic reasoning with statistical learning to provide AI that does not just calculate but actually gets it.
The Bigger Picture: Creating Context-Aware Intelligence
As artificial intelligence moves deeper into the programs we use, the systems we construct, and the choices we make, the demand for systems that comprehend context, subtlety, and human experience increases. Krishna Kumar's book is a persuasive addition to that imperative, a framework through which machines can do more than process information they can reason reasonable to manage the fine-grained logic that characterizes human intelligence.
Open in app